M. Sluydts
Race against the Machine: can deep learning recognize microstructures as well as the trained human eye?
Abstract
The promising results of deep learning in image recognition suggest a huge potential for microscopic analyses in materials science. One major challenge for its adoption in the study of materials is the limited number of images that are available to train models on. Herein, we present a methodology to create accurate image recognition models with small datasets. By explicitly taking into account the magnification and by introducing appropriate transformations, we incorporate as many insights from material science in the model as possible. This allows for a highly data-efficient training of complex deep learning models. Our results indicate that a model trained with the presented methodology is able to outperform human experts.
Compact representations of microstructure images using triplet networks
Abstract
The microstructure of a material, typically characterized through a set of microscopy images of two-dimensional cross-sections, is a valuable source of information about the material and its properties. Every pixel of the image is a degree of freedom causing the dimensionality of the information space to be extremely high. This makes it difficult to recognize and extract all relevant information from the images. Human experts circumvent this by manually creating a lower-dimensional representation of the microstructure. However, the question of how a microstructure image can be best represented remains open. From the field of deep learning, we present triplet networks as a method to build highly compact representations of the microstructure, condensing the relevant information into a much smaller number of dimensions. We demonstrate that these representations can be created even with a limited amount of example images, and that they are able to distinguish between visually very similar microstructures. We discuss the interpretability and generalization of the representations. Having compact microstructure representations, it becomes easier to establish processing–structure–property links that are key to rational materials design.

Mechanistic Insights in Seeded Growth Synthesis of Colloidal Core/Shell Quantum Dots
Abstract
We analyze the mechanism of seeded growth reactions used to synthesize colloidal core/shell nanocrystals. Looking at the formation of CdSe/CdS and CdSe/ZnSe using both zinc blende and wurtzite CdSe seeds with a different surface termination, we show that the formation rate of the shell material does not depend on the presence of the CdSe seed nanocrystals. This suggests that shells grow by inclusion of CdS or ZnSe initially formed in the reaction mixture, possibly under the form of reactive monomers, and not by successive adsorption and reaction of metal and chalcogen precursors. This insight makes balancing homogeneous nucleation and heterogeneous growth of the shell material key to suppressing spurious secondary nucleation. Through a combination of experimental work and reaction simulations, we show that this can be effectively achieved by raising the monomer solubility through the concentration of carboxylic acid used in the seeded growth reaction.
High-Throughput Screening of Extrinsic Point Defect Properties in Si and Ge: Database and Applications

Abstract
Increased computational resources now make it possible to generate large data sets solely from first principles. Such “high-throughput” screening is employed to create a database of embedding enthalpies for extrinsic point defects and their vacancy complexes in Si and Ge for 73 impurities from H to Rn. Calculations are performed both at the PBE and HSE06 levels of theory. The data set is verified by comparison of the predicted lowest-enthalpy positions with experimental observations. The effect of temperature on the relative occupation of defect sites is estimated through configurational entropy. Potential applications are demonstrated by selecting optimal vacancy traps, directly relevant to industrial processes such as Czochralski growth as a means to suppress void formation.
Error estimates for density-functional theory predictions of surface energy and work function
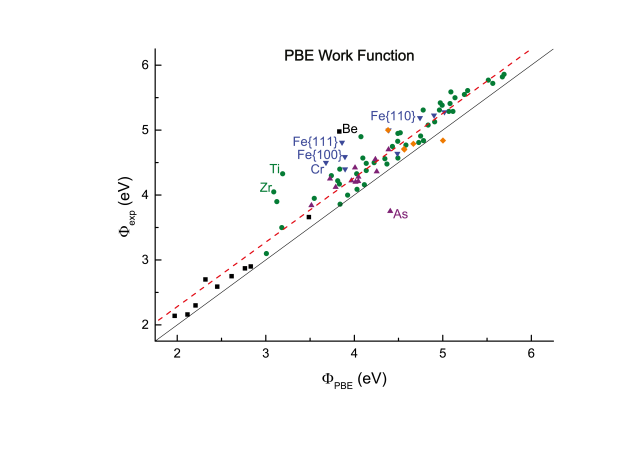
Abstract
Density-functional theory (DFT) predictions of materials properties are becoming ever more widespread. With increased use comes the demand for estimates of the accuracy of DFT results. In view of the importance of reliable surface properties, this work calculates surface energies and work functions for a large and diverse test set of crystalline solids. They are compared to experimental values by performing a linear regression, which results in a measure of the predictable and material-specific error of the theoretical result. Two of the most prevalent functionals, the local density approximation (LDA) and the Perdew-Burke-Ernzerhof parametrization of the generalized gradient approximation (PBE-GGA), are evaluated and compared. Both LDA and GGA-PBE are found to yield accurate work functions with error bars below 0.3 eV, rivaling the experimental precision. LDA also provides satisfactory estimates for the surface energy with error bars smaller than 10%, but GGA-PBE significantly underestimates the surface energy for materials with a large correlation energy.

Ligand Addition Energies and the Stoichiometry of Colloidal Nanocrystals
Abstract
Experimental non-stoichiometries of colloidal nanocrystals such as CdSe and PbS are accounted for by attributing to each constituent atom and capping ligand a formal charge equal to its most common oxidation state to obtain an overall neutral nanocrystal. In spite of its apparent simplicity, little theoretical support of this approach - called here the oxidation-number sum rule - is present in the current literature. Here, we introduce the ligand addition energy, which we define as the energy gained or expended upon the transfer of one ligand from a reference state to a (metal-rich) solid surface. For the combination of CdSe, ZnSe and InP with either chalcogen, halogen or hydrochalcogen ligands, we compute successive ligand addition energies using ab initio methods and determine the thermodynamically stable surface composition as that composition where ligand addition turns endothermic. We find that the oxidation-number sum rule is valid in many situations, although exceptions occur for each material studied most notably when exposed to small oxidative ligands. In the case of InP violations are more severe, extending towards the entire chalcogen ligand family. In addition, we find that electronegativity rather than chemical hardness is a reasonable predictor for ligand addition energies, with the most electronegative ligands yielding the most exothermic addition energies. Finally, we argue that the ligand addition energy will be a most useful quantity for future computational studies on the structure, stability and reactivity of nanocrystal surfaces.
Controlling the Size of Hot Injection Made Nanocrystals by Manipulating the Diffusion Coefficient of the Solute
Abstract
We investigate the relation between the chain length of ligands used and the size of the nanocrystals formed in the hot injection synthesis. With two different CdSe nanocrystal syntheses, we consistently find that longer chain carboxylic acids result in smaller nanocrystals with improved size dispersions. By combining a more in-depth experimental investigation with kinetic reaction simulations, we come to the conclusion that this size tuning is due to a change in the diffusion coefficient and the solubility of the solute. The relation between size tuning by the ligand chain length and the coordination of the solute by the ligands is further explored by expanding the study to amines and phosphine oxides. In line with the weak coordination of CdSe nanocrystals by amines, no influence of the chain length on the nanocrystals is found, whereas the size tuning brought about by phosphine oxides can be attributed to a solubility change. We conclude that the ligand chain length provides a practical handle to optimize the outcome of a hot injection synthesis in terms of size and size dispersion and can be used to probe the interaction between ligands and the actual solute.