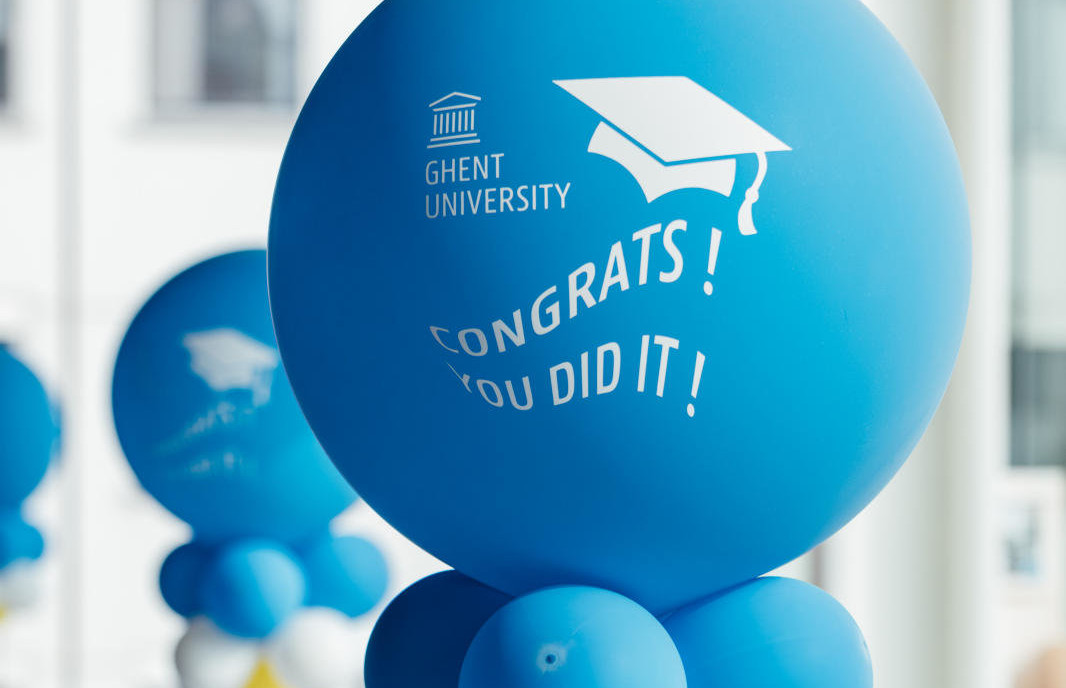
© UGent, foto Anneke D'Hollander
Last week two of our PhD fellows successfully defended their work. Congratulations to both!
Elias Van Den Broeck presented his work entitled ‘A Multiscale Modeling Approach to Understand Reactivity and Interactions in Complex Molecular Environments with Applications in Polymer Chemistry’ on Wednesday April 20th, 2022. He was supervised by prof. dr. ir. Veronique Van Speybroeck.
Summary
In this doctoral thesis, molecular modeling is employed to gain a fundamental understanding of reactivity and interactions in complex molecular environments. More specifically a broad range of modeling approaches is used to solve various scientific questions related to polymer chemistry, ranging from short to long timescales and from small to large scale systems. We show that molecular modeling is indeed an indispensible tool to understand polymerization features, governing molecular interactions, reaction mechanisms, reaction kinetics and material properties. Given the complexity of the studied systems, a complementary set of techniques is necessary to answer the scientific question at hand combining both static and dynamic approaches with classical and/or quantum mechanical models.
Additionally, to obtain an accurate description of the molecular system under investigation and its molecular environment, models need to account for the operating conditions such as realistic temperatures and a proper solvent environment. In this thesis we adopted, to a large extent, molecular dynamics (MD) simulations while explicitly considering the solvent environment. The work was performed in close collaboration with various experimental partners and to answer the scientific questions at hand, we had to apply a multiscale modeling approach. To this end, we have set up different protocols and workflows throughout this thesis in order to construct and equilibrate the molecular systems realistically and describe the corresponding chemistry in their complex molecular environments. Typically a trade-off is made between accuracy and computational cost when setting up the molecular model which inherently depends on the system under investigation and the scientific problem which has to be solved.
Leonid Komissarov worked under the supervision of prof. dr. ir. Toon Verstraelen. His dissertation entitled ‘Optimizing Potential Energy Surface Models’ was presented on Friday April 22nd, 2022.
Summary
The digital revolution has undoubtedly been a major contributor to the shaping of modern society. Nowadays computational simulations play an integral part in science and industry, enabling novel discoveries at an unprecedented pace. Such reliance on simulations means that there is a constant demand for hard- and software that produces results faster, more accurately and at a lower cost.
This thesis highlights a strategy that can deliver fast and accurate computational models: optimization; specifically in the context of empirical models of the potential energy surface, as used in physics and chemistry. Here, the problem of inaccurate predictions can be addressed by fitting model parameters to reference data. In doing so, a previously poor model can be trained to perform significantly better. Although this seems like a simple and viable approach on paper, the implementation is not straightforward: To date, there exists a plethora of models for molecular simulation, various optimization algorithms, and a number reference data sources. Until now the process of interfacing the above components has been tedious and prone to produce workflows that are of little comprehensive use to the scientific community.
We have developed a tool that alleviates these issues. It facilitates parameter optimization, allowing researchers to focus more on their science than the time-consuming technical details. In addition, the tool is highly flexible as users can mix and match various optimizers with different models and include any computable physicochemical property in the fitting procedure. The following pages provide an overview of how parametric molecular models can be optimized. We will discuss various types of models and optimization strategies before introducing our software tool. The advantages of our software are underlined with multiple successful parametrization examples. First applications of it resulted in improved performance of the ReaxFF and GFN1-xTB models. Both parametrizations have been made available to the scientific community and are discussed in the included papers.